ChatGPT,HuggingChat,Google Bard: Advanced Prompt Engineering

- Description
- Curriculum
- FAQ
- Reviews
Unlock the full potential of ChatGPT with our comprehensive course on “Mastering Prompt Engineering with ChatGPT”.
- Discover the secret techniques behind getting the most accurate answers from ChatGPT with our “Ultimate Guide to Prompt Engineering.” Say goodbye to frustrating misinterpretations and hello to optimized responses with our easy-to-follow course. Plus, no previous knowledge required.
- Are you tired of ChatGPT giving incorrect responses? Don’t waste any more time figuring things out by yourself! Our comprehensive course teaches you exactly what to do to maximize your results when using ChatGPT. Start creating professional applications today with ease.
- Take charge of ChatGPT and watch your career skyrocket with our one-of-a-kind course on “Prompt Engineering Fundamentals.” Learn insider tips, advanced techniques, and everything else you need to know to become an expert at asking the right questions of this revolutionary AI tool. Beginner friendly and results guaranteed. Sign up now before our next session fills up!
Our course is specifically designed to help you master the art of prompt engineering and get the best possible performance from ChatGPT.
In this course, you’ll learn the fundamentals of how to ask questions of ChatGPT and the technical term for it – Prompt Engineering. You’ll explore the applications of ChatGPT and gain knowledge on how to ask questions to optimize ChatGPT’s performance whatever profession you work in – be it writing emails, project management, marketing or development.
We’ll also cover the importance of context in prompt engineering and best practices for crafting effective prompts including the syntax of effective prompts. You’ll learn about common types of mistakes made by ChatGPT and how to avoid them.
Our course is ideal for students and professionals who want to master the art of prompt engineering with ChatGPT. No prior experience is necessary.
By the end of the course, you’ll be able to create effective prompts for ChatGPT and implement it in real-world applications. You’ll have a comprehensive understanding of prompt engineering and the power of AI. Join us on this exciting journey of mastering Prompt Engineering with ChatGPT!
-
1Introduction Video. Welcome!Video lesson
This course welcomes you to the course, gives you an introduction and also goes through what you will learn in this course including the different prompting techniques which will enhance your ability to use ChatGPT as well as the types of mistakes ChatGPT can make which you need to be aware of to make the best use of this tool.
Prompt Engineering: A Non-Technical Guide to ChatGPT is a course designed to help individuals learn how to create effective prompts for ChatGPT, the artificial intelligence chatbot developed by OpenAI. The course is suitable for anyone interested in learning how to use ChatGPT to generate human-like text and improve their personal and professional projects. The course covers the basics of prompt engineering, including prompt priming and how it can improve ChatGPT outputs. Participants will gain the confidence and know-how to use ChatGPT to its fullest potential and create their own custom prompts to enhance their personal and professional projects. The course includes a 17-page prompt engineering guide and a variety of prompts that can be used with ChatGPT. By the end of the course, participants will have the skills and knowledge to use ChatGPT to generate high-quality text and improve their projects.
-
2What this course coversText lesson
In this course on Prompt Engineering with ChatGPT, students will learn the following:
The history of Artificial Intelligence and its types.
How ChatGPT is made, its architecture, and applications.
The fundamentals of Natural Language Processing (NLP) and common mistakes in NLP.
How data is fed into ChatGPT and data pre-processing techniques.
The basics of Neural Networks and their types.
How to ask questions to get the best out of ChatGPT.
Importance of context in ChatGPT and best practices for prompt engineering.
Future of ChatGPT and Artificial Intelligence.
By the end of this course, learners will be able to achieve the following learning objectives:
Understand the basics of Artificial Intelligence and its types.
Understand the working of ChatGPT and its applications.
Gain knowledge of Natural Language Processing (NLP) and data pre-processing techniques.
Learn how to ask questions to get the best out of ChatGPT and how to prompt engineer effectively.
Be able to implement ChatGPT in real-world applications.
Be familiar with the future of ChatGPT and Artificial Intelligence.
Write who this course is designed for including any previous background required which should be none in bullet points
This course on Prompt Engineering with ChatGPT is designed for:
Students who want to learn about Artificial Intelligence and ChatGPT.
Professionals who want to implement ChatGPT in their work.
Enthusiasts who want to explore the world of Artificial Intelligence and ChatGPT.
No previous background is required to take this course. However, basic knowledge of programming and data analysis can be helpful.
-
3Introduction to ChatGPTVideo lesson
ChatGPT is an artificial intelligence language model that was developed by OpenAI, an artificial intelligence research organization. It is a neural network-based model that uses machine learning algorithms to generate human-like responses to text-based prompts.
The architecture of ChatGPT allows it to process text inputs, analyze the context, and generate outputs based on the learned patterns from large datasets. It can be used for a wide range of natural language processing tasks, such as language translation, text summarization, and conversational agents.
Unlike traditional search engines like Google, ChatGPT does not rely on specific keywords to generate responses. Instead, it understands the context of the text inputs and generates responses based on its learned patterns. ChatGPT can provide more personalized and contextualized responses to users, which can help to improve user experience.
Moreover, ChatGPT can learn and adapt to new data in real-time, which enables it to improve its performance continuously. This feature makes ChatGPT a powerful tool for businesses and individuals who want to interact with their customers in a more natural and engaging way.
In this course, we will explore the various applications of ChatGPT and how to implement it in real-world scenarios. We will also cover the common mistakes made in natural language processing and how to avoid them. So, let's dive into the exciting world of ChatGPT and unlock the power of artificial intelligence.
-
4Test your knowledgeQuiz
Test your knowledge
What is ChatGPT?
A. A virtual writing tutor
B. A customer support chatbot
C. A neural network-based language model
D. All of the above
Grade
Mark Complete
-
5Capabilities of ChatGPTText lesson
-
6Applications of ChatGPTText lesson
-
7What is Prompt Engineering?Video lesson
ChatGPT is an innovative conversational artificial intelligence (AI) system designed to produce human-like replies to natural language conversations between humans and machines. One of the essential aspects of ChatGPT is its ability to perform prompt engineering, which is a crucial process in conversational AI systems. This article will delve into prompt engineering, its importance, and how ChatGPT uses it to provide accurate responses.
Prompt engineering involves designing prompts, a set of questions, statements or responses a user can ask from an AI system such as ChatGPT. In other words, prompt engineering is about designing the prompts, training data, and building models that enable chatbots or virtual assistants to understand users' requests and provide prompt and accurate responses.
Prompts can vary from simple questions such as "What is your name?" to complex questions that require context, such as "Can you recommend a good Italian restaurant near me?" In conclusion, prompt engineering is an essential aspect of conversational AI systems such as ChatGPT.
A well-designed prompt can ensure that users are understood and provided with relevant and accurate responses. ChatGPT uses prompt engineering to train its model to understand natural language and recognize context, enabling it to provide meaningful human-like conversations with users. By understanding prompt engineering, students can appreciate the importance of prompt design in developing an intelligent conversational AI system like ChatGPT.
-
8What is Prompt EngineeringText lesson
-
9Elements of a PromptVideo lesson
Prompt engineering involves designing optimal prompts to instruct a language model to perform a specific task. To achieve the best results, prompts should contain certain elements that guide the model in generating the desired output. In this tutorial, we will go over the different components that make up a prompt.
Instruction The instruction is the specific task or action that you want the model to perform. It can be a question, a statement, or a command. For example, "summarize this article," "translate this sentence to Spanish," or "generate a creative story."
Context Context provides additional information that can steer the model to better responses. It can be external information, such as the topic of a conversation or the purpose of the task, or it can be information that the model has previously learned or generated. Context can help the model to understand the nuances of the input and generate a more accurate output.
Input Data Input data is the input or question that we are interested in finding a response for. It can be a sentence, a paragraph, a dataset, or any other type of input that the model can process. For example, "What is the capital of France?" or "Summarize this article about renewable energy."
Output Indicator The output indicator specifies the type or format of the output that we want the model to generate. It can be a specific type of output, such as a summary or a translation, or it can be a more general format, such as a paragraph or a list. The output indicator helps the model to understand the structure and format of the desired output.
Not all components are required for a prompt, and the format depends on the task at hand. Depending on the task, certain elements may be more important than others. For example, for a text classification task, the input data may be the most important element, while for a summarization task, the context and the output indicator may be crucial.
Basic Prompts
Let's start with a simple example of a basic prompt:
Prompt: The sky is
Output: blue
In this example, the language model outputs a continuation of strings that make sense given the context "The sky is". However, the output might be unexpected or far from the task we want to accomplish.
To improve the prompt, we can provide more context or instructions.
For example:
Prompt:
Complete the sentence: The sky is
Output:
so beautiful today.
Conclusion - Prompting is a powerful technique that allows us to instruct language models to perform specific tasks. By providing a well-crafted prompt, we can obtain better results from the model. With ChatGPT, we can easily prompt the model using the API and experiment with different prompts to achieve our desired results.
-
10General Tips for designing promptsVideo lesson
Tutorial: General Tips for Designing Prompts using ChatGPT
As you get started with designing prompts, it is essential to keep in mind that it is an iterative process that requires a lot of experimentation to get optimal results. Here are some tips to help you design effective prompts using ChatGPT:
Start Simple: Start with simple prompts and keep adding more elements and context as you aim for better results. Versioning your prompt along the way is vital for this reason. When you have a big task that involves many different subtasks, try to break down the task into simpler subtasks and keep building up as you get better results. This avoids adding too much complexity to the prompt design process at the beginning.
The Instruction: Use commands to instruct the model what you want to achieve, such as "Write," "Classify," "Summarize," "Translate," "Order," etc. Keep in mind that you need to experiment a lot to see what works best. Try different instructions with different keywords, contexts, and data and see what works best for your particular use case and task.
Specificity: Be very specific about the instruction and task you want the model to perform. The more descriptive and detailed the prompt is, the better the results. Providing examples in the prompt is very effective to get desired output in specific formats. However, including too many unnecessary details is not necessarily a good approach. The details should be relevant and contribute to the task at hand.
Avoid Impreciseness: It is essential to avoid imprecise descriptions in your prompt. Try to be specific and direct. The more direct, the more effective the message gets across. For example, when designing a prompt for a movie recommendation chatbot, instead of telling the model what not to do, it is better to focus on what it should do instead.
To Do or Not To Do? When designing prompts, it is crucial to focus on what to do instead of what not to do. This encourages more specificity and focuses on the details that lead to good responses from the model.
By following these tips, you can design effective prompts that produce the desired results. Remember that prompt design is an iterative process, and it takes a lot of experimentation to get optimal results.
-
11Examples of PromptsVideo lesson
Here are a few examples of prompts using ChatGPT :-
Summarize the following article on AI and its impact on the job market.
Write a short story about a time traveller who accidentally ends up in the wrong century.
Generate a list of 10 potential names for a new start-up company focused on renewable energy.
Translate the following sentence from French to English: "Je suis très heureux de vous rencontrer aujourd'hui."
Classify the following set of images into different categories: dogs, cats, birds, and fish.
Order the following list of events chronologically: the invention of the wheel, the discovery of electricity, and the first human spaceflight.
Generate a new recipe for a vegan lasagne using the following ingredients: eggplant, zucchini, tofu, spinach, and tomato sauce.
Write a poem about the beauty of nature and the changing seasons.
Provide a brief overview of the history and significance of the Great Wall of China.
Generate a new slogan for a brand of organic, fair-trade coffee.
-
12Best practices for Prompt EngineeringText lesson
-
13More Examples of PromptsVideo lesson
Prompts are used to instruct language models on what task to perform and how to perform it. There are several types of prompts, including text summarization, information extraction, question answering, text classification, conversation, code generation, and reasoning.
Text summarization prompts are used to generate quick and easy-to-read summaries of articles and concepts. An example of a text summarization prompt is asking the language model to explain antibiotics in one sentence.
Information extraction prompts are used to extract specific information from a given paragraph. An example of an information extraction prompt is asking the language model to mention the large language model based product mentioned in a given paragraph.
Question answering prompts are used to get the language model to respond to specific answers. A well-structured prompt that includes context, input, output, and instructions is essential for effective question answering. An example of a question answering prompt is asking the language model what OKT3 was originally sourced from.
Text classification prompts are used for harder use cases that require more context and elements to perform the task. These prompts require the prompt engineer to provide better instructions and think more about the context.
Here are examples of each type of prompt mentioned in the text:
Text Summarization: Prompt: Explain antibiotics Output: Antibiotics are a type of medication used to treat bacterial infections. They work by either killing the bacteria or preventing them from reproducing, allowing the body’s immune system to fight off the infection. Antibiotics are usually taken orally in the form of pills, capsules, or liquid solutions, or sometimes administered intravenously. They are not effective against viral infections, and using them inappropriately can lead to antibiotic resistance.
Prompt: Explain the above in one sentence Output: Antibiotics are medications used to treat bacterial infections by either killing the bacteria or stopping them from reproducing, but they are not effective against viruses and overuse can lead to antibiotic resistance.
Information Extraction: Prompt: Mention the large language model based product mentioned in the paragraph above Output: The large language model based product mentioned in the paragraph above is ChatGPT.
Question Answering: Prompt: Answer the question based on the context below. Keep the answer short and concise. Respond "Unsure about answer" if not sure about the answer. Context: Teplizumab traces its roots to a New Jersey drug company called Ortho Pharmaceutical. There, scientists generated an early version of the antibody, dubbed OKT3. Originally sourced from mice, the molecule was able to bind to the surface of T cells and limit their cell-killing potential. In 1986, it was approved to help prevent organ rejection after kidney transplants, making it the first therapeutic antibody allowed for human use. Question: What was OKT3 originally sourced from? Answer: Mice.
Text Classification: Prompt: Classify the sentiment of the following text as positive, negative, or neutral. Text: I had a great experience at the restaurant last night. The food was delicious and the service was excellent. Output: Positive.
Conversation: There isn't a specific example of a conversation prompt in the given text, but in general, a conversation prompt could be used to initiate a dialogue between a language model and a user, such as "Ask the model to recommend a book based on your interests" or "Have a conversation with the model about your favourite hobby."
Code Generation: There isn't a specific example of a code generation prompt in the given text, but in general, a code generation prompt could be used to instruct the language model to generate code based on a given input or task, such as "Write a Python function to calculate the mean of a list of numbers" or "Generate CSS code for a responsive navigation menu."
-
14Zero Shot PromptVideo lesson
Zero Shot Prompting: An Overview
Zero shot prompting is a recent development in natural language processing that allows language models to perform tasks without the need for task-specific training. Instead, a prompt is given to the language model, which includes instructions on what task to perform and how to perform it. This approach is particularly useful in situations where training data is limited or non-existent.
There are several types of prompts that can be used, including text summarization, information extraction, question answering, text classification, conversation, and code generation. Let's explore each of these types of prompts in more detail, along with some examples.
Text Summarization: Text summarization prompts are used to generate quick and easy-to-read summaries of articles and concepts. An example of a text summarization prompt is asking the language model to explain antibiotics in one sentence.
Information Extraction: Information extraction prompts are used to extract specific information from a given paragraph. An example of an information extraction prompt is asking the language model to mention the large language model based product mentioned in a given paragraph.
Question Answering: Question answering prompts are used to get the language model to respond to specific answers. A well-structured prompt that includes context, input, output, and instructions is essential for effective question answering. An example of a question answering prompt is asking the language model what OKT3 was originally sourced from.
Text Classification: Text classification prompts are used for harder use cases that require more context and elements to perform the task. These prompts require the prompt engineer to provide better instructions and think more about the context.
Conversation: Conversation prompts are used to initiate a dialogue between a language model and a user. For example, a conversation prompt could be used to ask the model to recommend a book based on the user's interests or to have a conversation with the model about the user's favorite hobby.
Code Generation: Code generation prompts are used to instruct the language model to generate code based on a given input or task. For example, a code generation prompt could be used to instruct the model to write a Python function to calculate the mean of a list of numbers or to generate CSS code for a responsive navigation menu.
Zero shot prompting has the potential to revolutionize natural language processing by allowing language models to perform tasks without the need for task-specific training. With the right prompts and instructions, language models can perform a wide range of tasks, from simple text summarization to complex code generation. As the field of natural language processing continues to evolve, we can expect to see even more exciting developments in the area of zero shot prompting.
-
15What is a Zero Shot PromptQuiz
This quiz looks at whether you understood what a zero shot prompt is
-
16Write 1 example of a zero shot promptText lesson
-
17Few Shot PromptVideo lesson
Few-shot prompting is a technique that is used to enable in-context learning in large language models. While zero-shot models are capable of handling simple tasks, they often fall short on more complex tasks. Few-shot prompting uses examples, or demonstrations, to condition the model for subsequent examples where the model is required to generate a response. The demonstrations act as conditioning, and they steer the model towards better performance.
The first appearance of few-shot prompting properties was when models were scaled to a sufficient size. According to Touvron et al. (2023), few-shot prompting has been used successfully with a 1-shot example. However, for more difficult tasks, increasing the demonstrations (e.g., 3-shot, 5-shot, 10-shot, etc.) can be experimented with.
The format used to present the examples also plays a crucial role in performance. Even if random labels are used, this is much better than no labels at all. Selecting random labels from a true distribution of labels instead of a uniform distribution also helps. The newer GPT models that are being experimented with are becoming more robust to even random formats.
Standard few-shot prompting works well for many tasks, but it still has limitations, especially when dealing with more complex reasoning tasks. Few-shot prompting is not enough to get reliable responses for this type of reasoning problem. The limitations of few-shot prompting demonstrate the need for more advanced prompt engineering.
Chain-of-thought (CoT) prompting has recently been popularized to address more complex arithmetic, common-sense, and symbolic reasoning tasks. CoT prompting breaks down the problem into steps and demonstrates them to the model.
In conclusion, few-shot prompting is a useful technique for solving some tasks. However, when zero-shot prompting and few-shot prompting are not sufficient, it might mean that a more advanced prompt engineering technique such as CoT prompting is required.
Here are a few examples of few-shot prompting:
Sentiment Analysis: Prompt: Positive This movie is amazing! This book was terrible! Negative I really enjoyed that play! Positive What a waste of money that was! Negative
Output: Positive Negative Positive Negative
In this example, we provide a few examples of positive and negative statements, along with the associated sentiment. The model is then able to classify the sentiment of other statements based on the few examples provided.
Text Generation: Prompt: Shakespeare's famous line "to be or not to be" can be completed with: A: that is the question. B: I am not sure. C: I like ice cream.
Output: that is the question.
In this example, we provide a few options to complete Shakespeare's famous line. The model is then able to generate the correct completion based on the few examples provided.
Question Answering: Prompt: Q: What is the capital of France? A: Paris Q: What is the largest continent? A: Asia Q: What is the highest mountain in the world? A: Output: Mount Everest
In this example, we provide a few questions and their corresponding answers. The model is then able to answer other questions based on the few examples provided.
These are just a few examples of few-shot prompting. The technique can be used for a variety of tasks and applications, and the number of examples provided can vary depending on the complexity of the task.
-
18One shot vs Few Shot PromptsQuiz
-
19Prompts for specificityQuiz
Which type of prompt is best suited for tasks that require a high degree of specificity? a) One shot prompts b) Few shot prompts
-
20Which type of prompt for training for a taskQuiz
If you want to train a model to recognize different types of flowers, which type of prompt would be most effective?
-
21Which type of prompt for complex tasksQuiz
Which type of prompt is more likely to result in accurate responses for complex tasks? a) One shot prompts b) Few shot prompts
-
22Which type of prompt for training a modelQuiz
If you want to train a model to recognize the emotions conveyed in text messages, which type of prompt would be most effective? a) One shot prompts b) Few shot prompts
-
23Chain of Thought PromptsVideo lesson
Chain-of-Thought Prompting: Improving Reasoning Capabilities
Chain-of-Thought (CoT) prompting is a technique that enables complex reasoning capabilities by breaking down a problem into intermediate reasoning steps. Introduced by Wei et al. in 2022, CoT prompting can be combined with few-shot prompting to improve results on more complex tasks that require reasoning before responding.
The idea behind CoT prompting is to provide a chain of reasoning steps that the language model can follow to arrive at the answer to a question. For instance, consider the following prompt:
"The odd numbers in this group add up to an even number: 4, 8, 9, 15, 12, 2, 1."
To answer this question, the language model needs to perform a series of intermediate reasoning steps, such as identifying the odd numbers in the group, adding them up, and determining whether the sum is even or odd. By providing these intermediate steps in the prompt, the language model can arrive at the answer more accurately.
The results of CoT prompting are impressive, as shown by the example above. By providing intermediate reasoning steps, the language model was able to accurately determine whether the odd numbers in the group add up to an even number, even when presented with different groups of numbers.
What's more, CoT prompting can be used with even fewer examples. The authors claim that just one example seems enough to solve some tasks. This is a promising development, as it means that CoT prompting can be used effectively even when there are not many examples available.
Zero-Shot CoT Prompting: Adding "Let's Think Step by Step"
Zero-shot CoT prompting is a more recent development that involves adding "Let's think step by step" to the original prompt. This technique was introduced by Kojima et al. in 2022 and is particularly useful when there are not many examples available.
Consider the following prompt:
"I went to the market and bought 10 apples. I gave 2 apples to the neighbour and 2 to the repairman. I then went and bought 5 more apples and ate 1. How many apples did I remain with?"
Without the "Let's think step by step" prompt, the language model might struggle to arrive at the correct answer. However, by providing a chain of reasoning steps, the language model can accurately determine the number of apples that remain.
The results of zero-shot CoT prompting are impressive, as shown by the example above. By providing intermediate reasoning steps, the language model was able to accurately determine the number of apples that remain, even when presented with a complex problem.
In conclusion, chain-of-thought prompting is a promising development in natural language processing. By breaking down a problem into intermediate reasoning steps, language models can arrive at more accurate answers, even when presented with complex tasks. Zero-shot CoT prompting is particularly useful when there are not many examples available, as it enables the language model to arrive at the correct answer with just a few prompts.
Sure, here are some examples of Chain of Thought (CoT) Prompting:
Math problems: Prompt: Solve the equation 2x + 3 = 7. Let's think step by step. Output: First, subtract 3 from both sides to get 2x = 4. Then, divide both sides by 2 to get x = 2.
Logical reasoning: Prompt: All dogs are animals. Some animals have fur. Therefore, some dogs have fur. Let's think step by step. Output: First, we know that all dogs are animals. Second, we know that some animals have fur. Therefore, it logically follows that some dogs have fur.
Science problems: Prompt: What is photosynthesis? Let's think step by step. Output: First, photosynthesis is the process by which plants convert light energy into chemical energy. Second, this process involves the absorption of light by pigments such as chlorophyll. Finally, this energy is used to synthesize organic molecules such as glucose from carbon dioxide and water.
Historical events: Prompt: What was the outcome of the Battle of Waterloo? Let's think step by step. Output: First, the Battle of Waterloo was fought between the French army led by Napoleon Bonaparte and the British army led by the Duke of Wellington. Second, the battle was fought near Waterloo, Belgium on June 18, 1815. Finally, the British army emerged victorious, effectively ending Napoleon's reign and leading to his exile to the island of Saint Helena.
These are just a few examples of how Chain of Thought Prompting can be used to enable complex reasoning and problem-solving capabilities.
-
24Self Consistency PromptText lesson
-
25General Knowledge PromptsText lesson
-
26Automatic Prompt EngineerText lesson
-
27Active PromptText lesson
-
28Applications of ChatGPT - ExamplesText lesson
-
29More applications of ChatGPTText lesson
-
30The Future of ChatGPTText lesson
-
31Advanced Prompt EngineeringText lesson
-
32How to give instructions to ChatGPTText lesson
-
33Learning Role PromptingText lesson
-
34Learning Few Shot PromptingText lesson
-
35Why we need Prompt PatternsText lesson
-
36Write 10 examples of Few Shot Prompting from your professionText lesson
-
37Meta Language PatternText lesson
-
38Error Resolution PatternText lesson
-
39Alternative Approaches PatternText lesson
-
40Explanation PatternText lesson
-
41Fact Checklist PatternText lesson
-
42Infinite Generation PatternText lesson
-
43Game Play PatternText lesson
-
44Refusal Breaker PatternText lesson
-
45Context Manager PatternText lesson
-
46Template PatternText lesson
-
47Alternative PatternText lesson
-
48Reflection PatternText lesson
-
49Recipe PatternText lesson
-
50Examples of Good Prompt EngineeringText lesson
-
51Prompt Pattern Catalog - IText lesson
A Prompt Pattern Catalog is a tool to enhance prompt engineering with large language models like ChatGPT. This catalog provides a standardized way to describe and categorize different types of prompts, making it easier for researchers and practitioners to understand and use them effectively.
Here are some examples of prompt patterns:
Role-Based Prompt:
You are a [role]. [Instruction/task]. [Context]. [Question]
Open-Ended Prompt:
[Context]. [Question]
Multiple-Choice Prompt:
[Context]. [Question]. [Option A]. [Option B]. [Option C]. [Option D].
Cloze Prompt:
[Context]. [Fill in the blank].
Yes/No Prompt:
[Context]. [Yes/No Question].
Conditional Prompt:
If [Condition], then [Instruction/task]. [Context]. [Question].
Comparison Prompt:
Compare [Object A] with [Object B]. [Context]. [Question].
Cause-and-Effect Prompt:
[Event A] caused [Event B]. [Context]. [Question].
Problem-Solving Prompt:
[Problem statement]. [Context]. [Question].
Few-Shot Learning Prompt:
[Example 1]. [Example 2]. [Example 3]. ... [Example N]. [Question].
These prompt patterns can be combined and customized in various ways to create prompts for specific tasks and domains. The Prompt Pattern Catalog serves as a reference for prompt engineering, allowing researchers and practitioners to experiment with different prompt patterns and optimize them for their specific use cases.
-
52Prompt Pattern Catalog - IIText lesson
-
53Understanding AI MindsText lesson
-
54IntroductionText lesson
-
55ACT LIKEText lesson
-
56INCLUDEText lesson
-
57FINDText lesson
-
58TRANSLATEText lesson
-
59DEFINEText lesson
-
60CONVERTText lesson
-
61CALCULATEText lesson
-
62GENERATEText lesson
-
63LISTText lesson
-
64CAUSEText lesson
-
65IMPACTText lesson
-
66REASONText lesson
-
67RECOMMENDText lesson
-
68EXPLAINText lesson
-
69OTHER PROMPT SYNTAX YOU CAN USEText lesson
-
70WITHOUTText lesson
-
71AND; OR; NOTText lesson
-
72Summarizing text with ChatGPTText lesson
-
73Structuring Data using ChatGPTText lesson
-
74Writing an email with ChatGPTText lesson
-
75Blog posts with ChatGPTText lesson
-
76Study Buddy with ChatGPTText lesson
-
77Coding Assistance with ChatGPTText lesson
-
78Generating Algorithms using ChatGPTText lesson
-
79Excel Formulas and Data Analytics using ChatGPTText lesson
-
80Multiple choice questions with ChatGPTText lesson
-
81Case studies on promptingText lesson
-
88History and Evolution of AIText lesson
-
89History of Neural NetworksText lesson
-
90History of Natural Language ProcessingText lesson
-
91History of Large Language ModelsText lesson
-
92How ChatGPT worksText lesson
-
93DAN and prompt hackingText lesson
-
94Jeoffrey Hinton's views on what AI can achieveText lesson
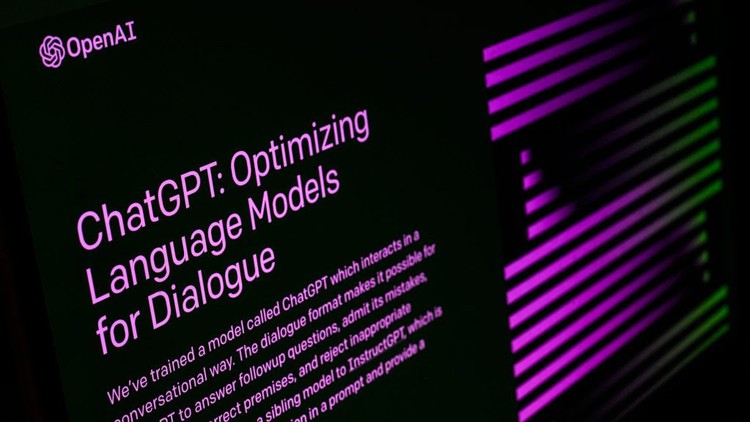
External Links May Contain Affiliate Links read more