2024 Python for Linear Regression in Machine Learning
- Description
- Curriculum
- FAQ
- Reviews
Unlock the power of machine learning with our comprehensive Python course on linear regression. Learn how to use Python to analyze data and build predictive models. This course is perfect for beginners with little or no programming experience and experienced Python developers looking to expand their skill set.
You’ll start with the basics of Python and work your way up to advanced techniques like linear regression, which is a powerful tool for predicting future outcomes based on historical data. Along the way, you’ll gain hands-on experience with popular Python libraries such as NumPy, Pandas, and Matplotlib. We will also cover the important aspect of model optimization, interpretability, and feature selection. You will learn how to optimize your model to improve its performance and how to interpret the model results and understand the underlying relationships in your data. We will also discuss feature selection techniques that are used to identify the most essential features that drive the predictions.
By the end of the course, you’ll have a solid understanding of how to use Python to build linear regression models and make accurate predictions. You’ll also be able to apply your new skills to a wide range of machine learning and data science projects. So, if you’re ready to take your Python skills to the next level and start using machine learning to analyze and predict real-world outcomes, this is the course for you!
What is covered in this course?
This course teaches you, step-by-step coding for Linear Regression in Python. The Linear Regression model is one of the widely used in machine learning and it is one the simplest ones, yet there is so much depth that we are going to explore in 14+ hours of videos.
Below are the course contents of this course:
-
Section 1- Introduction
This section gets you to get started with the setup. Download resources files for code along.
-
Section 2- Python Crash Course
This section introduces you to the basics of Python programming.
-
Section 3- Numpy Introduction
This section is optional, you may skip it but I would recommend you to watch it if you are not comfortable with NumPy.
-
Section 4- Pandas Introduction
This section introduces you to the basic concepts of Pandas. It will help you later in the course to catch up on the coding.
-
Section 5- Matplotlib Introduction
Do not skip this section. We will be using matplotlib plots extensively in the coming sections. It builds a foundation for a strong visualization of linear regression results.
-
Section 6- Linear Regression Introduction
We will kick-start our Linear Regression learning. You will learn the basics of linear regression. You will see some examples so that you can understand how Linear Regression works and how to analyze the results.
-
Section 7- Data Preprocessing for Linear Regression
This section is the most important section. DO NOT SKIP IT. It builds the foundation of data preprocessing for linear regression and other linear machine learning models. You will be learning, what are the techniques which we can use to improve the performance of the model. You will also learn how to check if your data is satisfying the coding of Linear Model Assumptions.
-
Section 8- Machine Learning Models Interpretability and Explainer
This section teaches you how to open-up any machine learning models. Now you don’t need to treat machine learning models as black-box, you will get to learn how to open this box and how to analyze each and every component of machine learning models.
-
Section 9- Linear Regression Model Optimization
This section extensively uses the knowledge of previous sections so don’t skip those. You will learn various techniques to improve model performance. We will show you how to do outliers removal and feature transformations.
-
Section 10- Feature Selection for Linear Regression
This section teaches you some of the best techniques of feature selection. Feature selection reduces the model complexity and chances of model overfitting. Sometimes the model also gets trained faster but mostly depends on how many features are selected and the types of machine learning models.
-
Section 11- Ridge & Lasso Regression, ElasticNet, and Nonlinear Regression
This section covers, various types of regression techniques. You will be seeing how to achieve the best accuracy by using the above techniques.
By the end of this course, your confidence will boost in creating and analyzing the Linear Regression model in Python. You’ll have a thorough understanding of how to use regression modeling to create predictive models and solve real-world business problems.
-
1IntroductionVideo lesson
I will cover regression from ground level to the advanced level.
-
2Resources Folder | DO NOT SKIP!Text lesson
-
3Install Anaconda and Python 3 on Windows 10Video lesson
-
4Install Anaconda and Python 3 on Ubuntu MachineVideo lesson
-
5Jupyter Notebook ShortcutsText lesson
-
6IntroductionVideo lesson
-
7Data TypesVideo lesson
-
8Variable AssignmentVideo lesson
-
9String AssignmentVideo lesson
-
10ListVideo lesson
-
11SetVideo lesson
-
12TupleVideo lesson
-
13DictionaryVideo lesson
-
14Boolean and Comparison OperatorVideo lesson
-
15Logical OperatorVideo lesson
-
16If, Else, ElifVideo lesson
-
17Loops in PythonVideo lesson
-
18Methods and Lambda FunctionVideo lesson
-
19IntroductionVideo lesson
-
20ArrayVideo lesson
-
21NaN and INFVideo lesson
-
22Statistical OperationsVideo lesson
-
23Shape, Reshape, Ravel, FlattenVideo lesson
-
24Sequence, Repetitions, and Random NumbersVideo lesson
-
25Where(), ArgMax(), ArgMin()Video lesson
-
26File Read and WriteVideo lesson
-
27Concatenate and SortingVideo lesson
-
28Working with DatesVideo lesson
-
37IntroductionVideo lesson
-
38Line PlotVideo lesson
-
39Label for X-Axis and Y-AxisVideo lesson
-
40Scatter Plot, Bar Plot, and Hist PlotVideo lesson
-
41Box PlotVideo lesson
-
42SubplotVideo lesson
-
43xlim, ylim, xticks, and yticksVideo lesson
-
44Pie PlotVideo lesson
-
45Pie Plot Text ColorVideo lesson
-
46Nested Pie PlotVideo lesson
-
47Labeling a Pie PlotVideo lesson
-
48Bar Chart on Polar AxisVideo lesson
-
49Line Plot on a Polar AxisVideo lesson
-
50Scatter Plot on a Polar AxisVideo lesson
-
51Integral in Calculus Plot as Area Under the CurveVideo lesson
-
52Linear Regression IntroductionVideo lesson
-
53Regression ExamplesVideo lesson
-
54Types of Linear RegressionVideo lesson
-
55Assessing the performance of the modelVideo lesson
-
56Bias-Variance tradeoffVideo lesson
-
57What is sklearn and train-test-splitVideo lesson
-
58Python Package Upgrade and ImportVideo lesson
-
59Load Boston Housing DatasetVideo lesson
-
60Dataset AnalysisVideo lesson
-
61Exploratory Data Analysis- Pair PlotVideo lesson
-
62Exploratory Data Analysis- Hist PlotVideo lesson
-
63Exploratory Data Analysis- HeatmapVideo lesson
-
64Train Test Split and Model TrainingVideo lesson
-
65How to Evaluate the Regression Model PerformanceVideo lesson
-
66Plot True House Price vs Predicted PriceVideo lesson
-
67Plotting Learning Curves Part 1Video lesson
-
68Plotting Learning Curves Part 2Video lesson
-
69Machine Learning Model Interpretability- Residuals PlotVideo lesson
-
70Machine Learning Model Interpretability- Prediction Error PlotVideo lesson
-
71Linear Model Assumption for Linear RegressionVideo lesson
-
72Definitions of Linear Model AssumptionsVideo lesson
-
73Load Boston DatasetVideo lesson
-
74Create Reference DataVideo lesson
-
75Check Linear Assumption for Boston Dataset Part 1Video lesson
-
76Check Linear Assumption for Boston Dataset Part 2Video lesson
-
77Log Transformation of VariablesVideo lesson
-
78Types of Variable TransformationsVideo lesson
-
79Reciprocal TransformationVideo lesson
-
80sqrt and exp TransformationVideo lesson
-
81Box-Cox TransformationVideo lesson
-
82Yeo-Johnson TransformationVideo lesson
-
83Check Variables Normality with HistogramVideo lesson
-
84Check Variables Normality with Q-Q PlotVideo lesson
-
85Variable Transformation for NormalityVideo lesson
-
86Check Variables HomocedasticityVideo lesson
-
87Variable Transformation for Homoscedasticity Part 1Video lesson
-
88Variable Transformation for Homoscedasticity Part 2Video lesson
-
89How to Check MulticolinearityVideo lesson
-
90Normalization and Standardization IntroductionVideo lesson
-
91Normalization and Standardization CodingVideo lesson
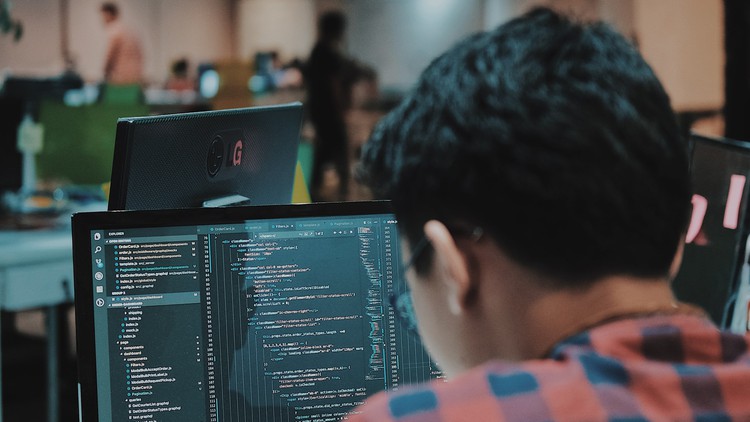
External Links May Contain Affiliate Links read more