Data Engineering Essentials - SQL, Python and Spark
- Description
- Curriculum
- FAQ
- Reviews
As part of this course, you will learn all the Data Engineering Essentials related to building Data Pipelines using SQL, Python as well as Spark.
About Data Engineering
Data Engineering is nothing but processing the data depending upon our downstream needs. We need to build different pipelines such as Batch Pipelines, Streaming Pipelines, etc as part of Data Engineering. All roles related to Data Processing are consolidated under Data Engineering. Conventionally, they are known as ETL Development, Data Warehouse Development, etc.
Course Details
As part of this course, you will be learning Data Engineering Essentials such as SQL, Programming using Python and Spark. Here is the detailed agenda for the course.
-
Database Essentials – SQL using Postgres
-
Getting Started with Postgres
-
Basic Database Operations (CRUD or Insert, Update, Delete)
-
Writing Basic SQL Queries (Filtering, Joins, and Aggregations)
-
Creating Tables and Indexes
-
Partitioning Tables and Indexes
-
Predefined Functions (String Manipulation, Date Manipulation, and other functions)
-
Writing Advanced SQL Queries
-
-
Programming Essentials using Python
-
Perform Database Operations
-
Getting Started with Python
-
Basic Programming Constructs
-
Predefined Functions
-
Overview of Collections – list and set
-
Overview of Collections – dict and tuple
-
Manipulating Collections using loops
-
Understanding Map Reduce Libraries
-
Overview of Pandas Libraries
-
Database Programming – CRUD Operations
-
Database Programming – Batch Operations
-
-
Setting up Single Node Cluster for Practice
-
Setup Single Node Hadoop Cluster
-
Setup Hive and Spark on Single Node Cluster
-
-
Introduction to Hadoop ecosystem
-
Overview of HDFS Commands
-
-
Data Engineering using Spark SQL
-
Getting Started with Spark SQL
-
Basic Transformations
-
Managing Tables – Basic DDL and DML
-
Managing Tables – DML and Partitioning
-
Overview of Spark SQL Functions
-
Windowing Functions
-
-
Data Engineering using Spark Data Frame APIs
-
Data Processing Overview
-
Processing Column Data
-
Basic Transformations – Filtering, Aggregations, and Sorting
-
Joining Data Sets
-
Windowing Functions – Aggregations, Ranking, and Analytic Functions
-
Spark Metastore Databases and Tables
-
Desired Audience
Here is the desired audience for this course.
-
College students and entry-level professionals to get hands-on expertise with respect to Data Engineering. This course will provide enough skills to face interviews for entry-level data engineers.
-
Experienced application developers to gain expertise related to Data Engineering.
-
Conventional Data Warehouse Developers, ETL Developers, Database Developers, PL/SQL Developers to gain enough skills to transition to be successful Data Engineers.
-
Testers to improve their testing capabilities related to Data Engineering applications.
-
Any other hands-on IT Professional who wants to get knowledge about Data Engineering with Hands-On Practice.
Prerequisites
-
Logistics
-
Computer with decent configuration (At least 4 GB RAM, however 8 GB is highly desired)
-
Dual Core is required and Quad-Core is highly desired
-
Chrome Browser
-
High-Speed Internet
-
-
Desired Background
-
Engineering or Science Degree
-
Ability to use computer
-
Knowledge or working experience with databases and any programming language is highly desired
-
Training Approach
Here are the details related to the training approach.
-
It is self-paced with reference material, code snippets, and videos provided as part of Udemy.
-
One can either use the environment provided by us or set up their own environment using Docker on AWS or GCP or the platform of their choice.
-
We would recommend completing 2 modules every week by spending 4 to 5 hours per week.
-
It is highly recommended to take care of the exercises at the end to ensure that you are able to meet all the key objectives for each module.
-
Support will be provided either through Udemy Q&A.
Self Evaluation
The course is designed in such a way that one can self-evaluate through the course and confirm whether the skills are acquired.
-
Here is the approach we recommend you to take this course.
-
The course is hands-on, you should practice as you go through the course.
-
You should also spend time understanding the concepts. If you do not understand the concept, I would recommend moving on and come back later to the topic.
-
Go through the consolidated exercises and see if you are able to solve the problems or not.
-
Make sure to follow the order we have defined as part of the course.
-
After each and every section or module, make sure to solve the exercises. We have provided enough information to validate the output.
-
-
By the end of the course, then you can come to the conclusion that you are able to master essential skills related to SQL, Python, and Spark.
-
11Introduction - Setup Postgres DatabaseVideo lesson
-
12Setup Postgres using DockerVideo lesson
-
13Docker Cheat SheetVideo lesson
-
14Accessing Postgres using Docker CLIVideo lesson
-
15Create Database and UserVideo lesson
-
16Execute SQL ScriptsVideo lesson
-
17SQL Workbench and PostgresVideo lesson
-
18Jupyter Lab and PostgresqlVideo lesson
-
19Connecting to DatabaseVideo lesson
-
20Using psqlVideo lesson
-
21Setup Postgres using DockerVideo lesson
-
22Setup SQL WorkbenchVideo lesson
-
23SQL Workbench and PostgresVideo lesson
-
24SQL Workbench FeaturesVideo lesson
-
25Data Loading UtilitiesVideo lesson
-
26Loading Data - DockerVideo lesson
-
35Standard TransformationsVideo lesson
-
36Overview of Data ModelVideo lesson
-
37Define Problem StatementVideo lesson
-
38Preparing TablesVideo lesson
-
39Selecting or Projecting DataVideo lesson
-
40Filtering DataVideo lesson
-
41Joining Tables - InnerVideo lesson
-
42Joining Tables - OuterVideo lesson
-
43Performing AggregationsVideo lesson
-
44Sorting DataVideo lesson
-
45Solution - Daily Product RevenueVideo lesson
-
46Exercises - Writing Basic SQL QueriesVideo lesson
-
47DDL - Data Definition LanguageVideo lesson
-
48Overview of Data TypesVideo lesson
-
49Adding or Modifying ColumnsVideo lesson
-
50Different Type of ConstraintsVideo lesson
-
51Managing ConstraintsVideo lesson
-
52Indexes on TablesVideo lesson
-
53Indexes for ConstraintsVideo lesson
-
54Overview of SequencesVideo lesson
-
55Truncating TablesVideo lesson
-
56Dropping TablesVideo lesson
-
57Overview of PartitioningVideo lesson
-
58List PartitioningVideo lesson
-
59Managing Partitions - ListVideo lesson
-
60Manipulating DataVideo lesson
-
61Range PartitioningVideo lesson
-
62Managing Partitions - RangeVideo lesson
-
63Repartitioning - RangeVideo lesson
-
64Hash PartitioningVideo lesson
-
65Managing Partitions - HashVideo lesson
-
66Usage ScenariosVideo lesson
-
67Sub PartitioningVideo lesson
-
68Exercise - Partitioned TablesVideo lesson
-
69Overview of FunctionsVideo lesson
-
70String Manipulation FunctionsVideo lesson
-
71Case Conversion and LengthVideo lesson
-
72Extracting Data - Using substr and split_partVideo lesson
-
73Using position or strposVideo lesson
-
74Trimming and Padding FunctionsVideo lesson
-
75Reverse and Concatenate Multiple StringsVideo lesson
-
76String ReplacementVideo lesson
-
77Date Manipulation FunctionsVideo lesson
-
78Getting Current Date or TimestampVideo lesson
-
79Date ArithmeticVideo lesson
-
80Beginning Date or Time using date_truncVideo lesson
-
81Using to_char and to_dateVideo lesson
-
82Extracting Information using extractVideo lesson
-
83Dealing with Unix Timestamp or epochVideo lesson
-
84Overview of Numeric FunctionsVideo lesson
-
85Data Type ConversionVideo lesson
-
86Handling NULL ValuesVideo lesson
-
87Using CASE and WHENVideo lesson
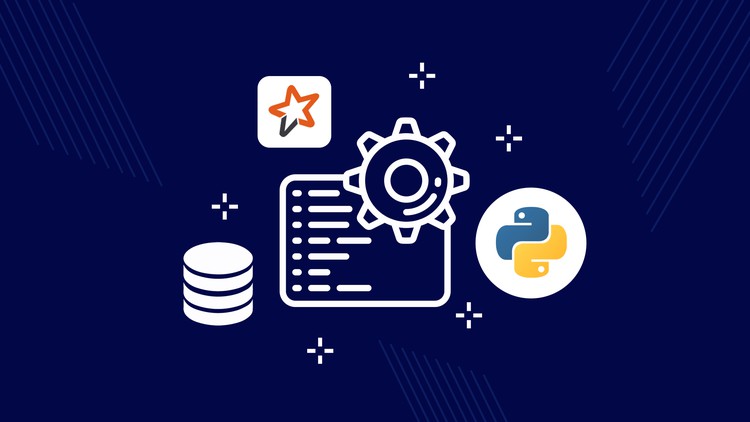
External Links May Contain Affiliate Links read more