Mastering AI in Operations
- Description
- Curriculum
- FAQ
- Reviews
Mastering AI in Operations: From Supply Chain to Workforce Optimization**
Are you ready to unlock the power of artificial intelligence to transform your operations? In today’s fast-paced business environment, organizations that embrace AI are positioned to gain a competitive edge, streamline processes, and maximize efficiency. Whether you’re an operations manager or a business leader, this course will equip you with the tools and strategies you need to harness AI’s potential and apply it directly to your operations.
**”Mastering AI in Operations: From Supply Chain to Workforce Optimization”** is designed to help you integrate AI into every aspect of your operations—from optimizing your supply chain to predicting workforce needs. With practical insights and real-world case studies, this course will show you how leading companies are leveraging AI to improve decision-making, reduce costs, and enhance overall efficiency.
### **What You’ll Learn**
This comprehensive course covers six key areas where AI can revolutionize your operations:
1. **AI-Driven Supply Chain Optimization**: Learn how to improve supply chain efficiency with AI-powered predictive analytics. You’ll discover how companies like Siemens and DHL use AI to forecast demand, monitor real-time data, and proactively manage disruptions. You’ll walk away with actionable strategies to enhance your own supply chain processes.
2. **Predictive Maintenance & Asset Management**: Discover how AI is transforming asset management by predicting equipment failures before they occur. Explore how companies like General Motors and Rolls-Royce have saved millions by avoiding costly downtime. This module will teach you how to implement AI-driven maintenance in your operations, reducing repair costs and extending the life of your assets.
3. **AI and Demand Forecasting**: Gain a deep understanding of how AI improves demand forecasting by analyzing vast datasets, including customer behavior, weather patterns, and social media trends. Companies like Procter & Gamble and Zara use AI to predict demand accurately—now, you can too.
4. **AI-Enhanced Quality Control**: Learn how to use AI to improve product quality by detecting defects that human inspectors might miss. We’ll explore how businesses like Audi and Pfizer are using AI to ensure their products meet the highest quality standards, reducing rework and enhancing customer satisfaction.
5. **Workforce Management with AI**: Optimize your workforce with AI-powered scheduling, productivity tracking, and performance analysis. Discover how companies like Amazon and Hilton are using AI to predict staffing needs and improve employee efficiency.
6. **Future-Proofing Your Workforce**: Understand how AI can help you stay ahead of industry trends, predict workforce needs, and upskill your employees. Learn how AI can be integrated into your long-term workforce strategy to ensure your team remains agile and competitive.
### **Why Take This Course?**
This course is designed for **operations managers, business leaders, and anyone interested in using AI to drive operational excellence**. You’ll gain a deep understanding of AI’s practical applications, and by the end of the course, you’ll be ready to implement AI-driven strategies that can transform your operations.
With **real-world examples, practical tools**, and **step-by-step guidance**, this course offers everything you need to begin your AI journey. Ready to optimize your operations with AI? Enroll today and start mastering the future of business operations!
-
3Unit 1, Lecture 1: The Future of Operations: Why AI MattersVideo lesson
### **Unit 1, Lecture 1: The Future of Operations: Why AI Matters**
Welcome to the first lecture in this course. Today, we’re diving into why AI matters in operations and how it's already transforming industries across the globe. Now, you might be thinking, “AI is just for tech giants like Google or Amazon, right?” Wrong. AI is reshaping operations in companies of all sizes—from local manufacturers to multinational corporations.
Let’s start with a story.
In 2015, Unilever, the global consumer goods company, faced significant challenges in their supply chain. Managing inventory for thousands of products across multiple regions was becoming a nightmare. They turned to AI for help. By integrating AI-powered demand forecasting tools, Unilever was able to cut inventory holding by 15% while improving service levels. These AI systems predicted product demand more accurately, ensuring that factories produced the right amount at the right time.
AI matters because it allows businesses to optimize processes in ways humans cannot. It can identify patterns, handle massive datasets, and make predictions at a speed and scale that is impossible for even the most skilled human operator. In the world of operations, where efficiency is everything, this is a game-changer.
But it’s not just about numbers and data. AI brings transformative potential in decision-making. Let’s consider Walmart, which uses AI to streamline its supply chain. AI helps Walmart manage over 11,000 stores worldwide, predicting which products will sell in specific locations, at specific times. This isn’t just improving efficiency—it’s driving better customer satisfaction and increasing sales by ensuring products are available exactly when customers need them.
By the end of this course, you’ll understand how AI can give your operations a competitive edge, regardless of your company size. Whether it’s predicting machine failures, optimizing your workforce, or improving your supply chain, AI offers a path to higher efficiency, lower costs, and better decision-making.
In the next lecture, we’ll explore the basics of AI and machine learning, so you can understand the core technologies driving these innovations.
---
-
4Unit 1, Lecture 2: Understanding the Basics of AI and Machine LearningVideo lesson
### **Unit 1, Lecture 2: Understanding the Basics of AI and Machine Learning**
Now that we understand why AI is so critical, let’s break down what AI actually is. You’ve probably heard terms like machine learning, deep learning, and automation—but what do they mean, and how do they apply to operations?
At its core, **AI** refers to machines designed to simulate human intelligence. **Machine learning** is a specific branch of AI that allows machines to learn from data. Rather than being explicitly programmed for every single task, machine learning models use data to improve over time.
Here’s a simple analogy: Think of machine learning like teaching a child to recognize dogs. At first, you show the child hundreds of pictures of different dogs. Over time, they start recognizing dogs without being told. The more dogs they see, the better they get at identifying one. That’s what machine learning does, but with data.
For example, UPS, the global logistics company, uses AI and machine learning to optimize its delivery routes. Instead of manually plotting every route, they feed their machine learning models with millions of data points—traffic patterns, weather conditions, delivery times—and the system suggests the most efficient routes. As UPS gathers more data, the AI improves, saving the company time, fuel, and money. It’s estimated that this AI-driven approach has saved UPS over 10 million gallons of fuel annually.
Another key concept is **automation**. AI can automate repetitive tasks, freeing up human resources for more complex decision-making. For instance, Amazon uses AI-powered robots in its warehouses to sort, pick, and move items. While humans still oversee operations, these robots handle the heavy lifting and repetitive tasks, speeding up the fulfillment process.
By now, you should be getting a clearer picture of how AI and machine learning work. In the next lecture, we’ll explore why integrating AI into your operations isn’t just a tech trend, but a business imperative for achieving better results.
---
-
5Unit 1, Lecture 3: The Business Case for AI in OperationsVideo lesson
### **Unit 1, Lecture 3: The Business Case for AI in Operations**
So far, we’ve explored the transformative potential of AI and the core technologies behind it. But the real question is, why should you—an operations manager or business leader—invest in AI? What’s the return on investment, and how do we justify it?
Let’s start with a case study from General Electric (GE). In the energy sector, GE employs AI to predict equipment failures in their wind turbines. Using data from sensors, their AI system can predict when a turbine is likely to fail, allowing them to carry out preventative maintenance. Before AI, GE was forced to wait for turbines to break down before making repairs, leading to costly downtime. Now, they predict failures and fix them before they occur. This has resulted in millions of dollars in savings due to reduced downtime and improved efficiency.
AI isn’t just about reducing costs; it’s about increasing efficiency and creating value. Consider Starbucks, which uses AI to personalize customer experiences. They analyze data on purchase history, location, and even weather conditions to suggest the right product to each customer at the right time. This personalization boosts customer satisfaction and drives repeat business.
The business case for AI is strong because it enables better decision-making. In operations, you’re constantly faced with choices—what to produce, when to produce, how much to store, when to repair, and more. AI helps you make smarter, faster decisions by analyzing massive amounts of data and identifying patterns that humans might miss. It reduces uncertainty and improves accuracy, leading to more efficient operations overall.
But let’s not forget about the ethical considerations. With AI making more decisions, it’s crucial to build systems that are fair, transparent, and accountable. Companies like Google are at the forefront of this, using AI in their operations while ensuring it aligns with ethical standards. For example, Google is investing heavily in explainable AI, ensuring that the decisions AI makes can be understood and justified by human operators.
To summarize, the business case for AI is not just about reducing operational costs; it’s about improving efficiency, creating value, and making more informed decisions. In the following units, we’ll dive deeper into specific applications of AI, starting with supply chain optimization.
---
-
6Unit 2, Lecture 1: Enhancing Supply Chain Efficiency with AIVideo lesson
### **Unit 2, Lecture 1: Enhancing Supply Chain Efficiency with AI**
Welcome to Unit 2, where we will explore the transformative power of AI in supply chain optimization. Supply chains are the backbone of any business, ensuring that products move efficiently from production to consumers. However, supply chains are also complex, often spanning multiple regions and involving countless variables. This is where AI steps in.
Let’s start with an example from **Siemens**, a company that uses AI to optimize its global supply chain. Siemens manufactures electrical and electronic products across 190 countries, which means their supply chain is vast and intricate. By implementing AI-powered predictive analytics, Siemens streamlined its logistics, ensuring the timely delivery of components to production sites. These AI systems help predict potential bottlenecks and recommend solutions to avoid them, reducing delays by 20%.
AI enhances supply chain efficiency in a few key ways:
1. **Real-time Data Monitoring**: Traditional supply chains relied on static data, often making decisions based on outdated information. AI, however, uses real-time data—whether it’s traffic updates, weather changes, or even social media trends—to adjust routes and logistics in real-time. This allows companies to respond swiftly to changes.
2. **Predictive Analytics**: With the help of machine learning algorithms, AI predicts demand fluctuations, potential disruptions, and even inventory needs, allowing businesses to preemptively adjust their supply chain strategies.
Let’s take another example—**DHL**, the global logistics giant. They use AI to monitor hundreds of variables, from road conditions to vehicle health, in order to improve route efficiency. DHL’s AI system recommends optimal routes for delivery trucks, reducing fuel consumption and cutting delivery times by up to 15%. That’s a significant improvement for a company that manages millions of deliveries each year.
By the end of this unit, you’ll understand how AI can boost your supply chain’s efficiency, making it more resilient and responsive to changes. In the next lecture, we’ll focus on predictive analytics, which is one of the most powerful applications of AI in supply chain optimization.
---
-
7Unit 2, Lecture 2: Leveraging Predictive Analytics for Supply Chain SuccessVideo lesson
### **Unit 2, Lecture 2: Leveraging Predictive Analytics for Supply Chain Success**
In the previous lecture, we touched on how AI enhances supply chain efficiency through real-time monitoring and predictive analytics. Today, we’ll dive deeper into predictive analytics and explore how it can be the difference between a reactive supply chain and a proactive one.
Predictive analytics involves using AI algorithms to forecast future events based on historical data. This can mean anything from predicting product demand, to foreseeing potential delays due to weather, to estimating when equipment in your supply chain might break down.
Take the case of **Procter & Gamble (P&G)**, the global consumer goods company. P&G uses AI-driven predictive analytics to forecast demand for its products. By analyzing vast amounts of historical data—including customer preferences, seasonal trends, and competitor activity—P&G’s AI system can predict which products will be in high demand in specific regions. This helps P&G adjust its production levels accordingly, avoiding both stock shortages and overproduction.
The real advantage of predictive analytics is that it moves your supply chain from reactive to proactive. Traditionally, supply chains have always responded to disruptions after they happen. If a shipment is delayed due to bad weather, for example, contingency plans are made after the fact. But with AI, predictive models can anticipate these disruptions before they occur. This gives operations teams time to respond in advance, potentially saving millions in lost revenue.
Another great example is **Zara**, the fast-fashion retailer. Zara uses AI to predict fashion trends and adjust its production and distribution accordingly. In the highly volatile fashion industry, predicting consumer demand can be difficult. But Zara’s AI system analyzes customer data, social media trends, and even influencer activity to forecast which items will be popular. As a result, Zara is able to get the right products to the right stores at the right time, minimizing waste and maximizing profits.
By leveraging predictive analytics, companies can gain a competitive edge. Not only does it enhance decision-making, but it also reduces costs associated with overproduction, delays, and inefficiencies. In the next lecture, we’ll look at real-world case studies where AI-driven supply chains have achieved remarkable results.
---
-
8Unit 2, Lecture 3: Real-World AI in Supply Chain: Case StudiesVideo lesson
### **Unit 2, Lecture 3: Real-World AI in Supply Chain: Case Studies**
In this lecture, we’re going to explore some real-world examples of how companies have successfully integrated AI into their supply chains. These case studies will show you just how impactful AI can be when applied strategically.
Let’s start with **Amazon**, a company that’s become a global leader largely because of its AI-powered supply chain. Amazon’s logistics network is massive, processing billions of orders each year. To manage this, Amazon uses AI to predict demand, optimize warehouse operations, and even route deliveries. One of Amazon’s key innovations is its AI-driven forecasting system, which predicts customer demand with incredible accuracy. By doing so, Amazon can stock its warehouses with the right products, ensuring faster delivery times and reducing the need for excess inventory.
But Amazon’s AI goes even further. In their fulfillment centers, AI-powered robots pick and move products, while machine learning algorithms constantly optimize warehouse layouts for maximum efficiency. This seamless integration of AI has allowed Amazon to deliver products faster and more cost-effectively than its competitors, setting a new standard for global supply chains.
Now, let’s look at **Coca-Cola**, another industry leader that’s using AI in innovative ways. Coca-Cola operates one of the world’s largest beverage supply chains, serving over 200 countries. They’ve implemented AI to predict inventory needs in their distribution centers. By using machine learning to analyze sales data, weather conditions, and even sporting events, Coca-Cola can predict which products will be in demand at different times of the year. This ensures that their distribution centers are always stocked with the right amount of product, reducing waste and optimizing storage space.
Coca-Cola has also applied AI to their bottling operations, using predictive maintenance to ensure that their equipment is always running smoothly. AI systems monitor the performance of bottling machines in real-time, predicting when a breakdown might occur. This allows Coca-Cola to perform maintenance before any issues arise, avoiding costly downtime.
Finally, let’s consider **IBM**, which has transformed its own supply chain using AI. IBM’s AI system, Watson, analyzes massive datasets to improve demand forecasting, manage supplier relationships, and monitor production schedules. IBM’s AI doesn’t just stop at predictive analytics—it also provides recommendations to supply chain managers, suggesting actions to prevent potential issues. This proactive approach has significantly reduced IBM’s supply chain disruptions, improving their ability to meet customer demand.
These examples show that AI isn’t just a tool for improving efficiency—it’s a strategic advantage that can revolutionize the way supply chains operate. Whether you’re optimizing for speed, cost, or customer satisfaction, AI has the power to elevate your supply chain to new heights.
In our next unit, we’ll dive into how AI can be applied to predictive maintenance and asset management, ensuring that your equipment runs smoothly and efficiently.
---
-
9Unit 3, Lecture 2: Implementing AI for Equipment Health MonitoringVideo lesson
### **Unit 3, Lecture 2: Implementing AI for Equipment Health Monitoring**
Now that we’ve explored the power of predictive maintenance, let’s discuss how you can implement AI-driven systems to monitor the health of your equipment. It’s important to understand that predictive maintenance isn’t just about predicting when something will break; it’s about understanding the health of your assets in real-time.
One of the key technologies behind predictive maintenance is **IoT (Internet of Things)**. IoT devices, like sensors, are used to collect data on equipment performance—temperature, vibration, pressure, and more. This data is then analyzed by AI models, which can identify patterns that indicate potential failures.
For example, **Boeing** uses IoT sensors and AI to monitor their aircraft engines and other critical components. These sensors gather data on engine vibrations, fuel usage, and other factors during flights. The AI analyzes this data in real-time, detecting any anomalies that may signal an issue. Boeing’s system can then alert maintenance crews to address the problem before it causes any serious disruptions. This has significantly reduced maintenance-related delays for their fleet.
But how do you get started with AI-powered health monitoring in your operations? Here’s a step-by-step approach:
1. **Identify Key Assets**: Start by identifying the most critical equipment in your operation. These are the assets where failure would cause the most significant downtime or financial loss.
2. **Install Sensors**: Equip these assets with IoT sensors that can monitor relevant metrics, such as temperature, vibration, or pressure.
3. **Data Collection**: Use AI systems to collect and analyze the data from these sensors. This data will help the AI model “learn” what normal operation looks like and recognize when something is off.
4. **Predictive Models**: Use machine learning models to predict when a failure is likely to occur. These models improve over time as they analyze more data, becoming more accurate in their predictions.
Let’s consider **Daimler Trucks North America (DTNA)**, which uses AI-powered health monitoring for its fleet of trucks. They have installed IoT sensors in key areas like the engine, transmission, and brakes to monitor performance. The AI system analyzes the data from these sensors, predicting when a truck will need maintenance. This allows DTNA to schedule maintenance before a breakdown occurs, minimizing costly repairs and improving fleet uptime.
AI-powered health monitoring ensures that your equipment runs smoothly and that you only perform maintenance when it’s needed. This helps avoid unnecessary maintenance costs and keeps operations running efficiently. In the next lecture, we’ll look at more case studies from companies that have successfully implemented predictive maintenance to transform their operations.
-
10Unit 3, Lecture 3: Case Studies in AI-Driven Predictive MaintenanceVideo lesson
### **Unit 3, Lecture 3: Case Studies in AI-Driven Predictive Maintenance**
In this final lecture of Unit 3, we’ll explore more real-world examples of companies that have successfully implemented AI-driven predictive maintenance. These case studies demonstrate the tangible benefits of predictive maintenance, from cost savings to improved operational efficiency.
Let’s start with **Siemens**, the global industrial manufacturing company. Siemens uses AI to monitor the health of its gas turbines. These turbines are complex pieces of machinery that power electricity plants worldwide. A failure in one of these turbines could lead to costly downtime for both Siemens and its customers. To prevent this, Siemens installed IoT sensors on the turbines to collect data on their performance—everything from temperature and pressure to fuel efficiency. The AI system analyzes this data in real-time and predicts when a part is likely to fail. This has allowed Siemens to reduce turbine downtime by 50% and has saved millions of dollars in repair costs.
Another example comes from **Union Pacific Railroad**, one of the largest freight railroad networks in the U.S. Union Pacific uses AI to monitor the performance of its trains and rail infrastructure. By installing sensors on train tracks and locomotives, they can gather data on things like track vibration, train speed, and wheel performance. The AI system analyzes this data and predicts when track maintenance or repairs are needed. Before AI, Union Pacific had to rely on scheduled maintenance checks, which often missed underlying issues. Now, with predictive maintenance, they’ve reduced derailments by 75%, improving both safety and operational efficiency.
Next, let’s look at **Shell**, the multinational oil and gas company. Shell operates oil rigs and refineries around the world, where equipment failure can lead to massive operational disruptions. Shell implemented AI-driven predictive maintenance across its refineries, monitoring everything from pumps to pipelines. The AI system uses sensor data to predict when equipment will need repairs, allowing Shell to fix issues before they cause a breakdown. This has led to a 20% reduction in maintenance costs and has significantly improved the safety of their operations.
These examples illustrate the transformative power of AI in predictive maintenance. Whether you’re managing industrial machinery, a fleet of vehicles, or a complex infrastructure, AI can help you monitor equipment health, predict failures, and ensure that your assets are operating at peak efficiency.
As we conclude this unit, it’s clear that predictive maintenance is more than just a cost-saving measure—it’s a strategic advantage. By adopting AI, companies can avoid costly downtime, extend the lifespan of their assets, and optimize their operations for long-term success.
In our next unit, we’ll dive into another exciting application of AI: demand forecasting. We’ll explore how AI can predict customer demand, helping businesses optimize production, inventory, and distribution strategies.
---
-
11Unit 4, Lecture 1: Forecasting Demand with AI: An OverviewVideo lesson
### **Unit 4, Lecture 1: Forecasting Demand with AI: An Overview**
Welcome to Unit 4. Today, we’re exploring how AI is transforming demand forecasting, an essential function in operations management. Predicting demand accurately is critical for maintaining efficient inventory, optimizing production, and ensuring customer satisfaction. However, traditional forecasting methods often rely on historical data and manual processes, which can’t always keep up with real-time changes in customer behavior or external factors like economic shifts.
This is where AI steps in, offering more precise, real-time demand forecasting. AI doesn’t just look at historical sales data—it analyzes numerous variables like weather patterns, social media trends, competitor actions, and economic indicators to make highly accurate predictions.
Let’s start with an example from **H&M**, the global fashion retailer. The fashion industry is notoriously fast-paced, and predicting customer demand is challenging. In the past, H&M would manually analyze historical sales data to forecast demand for upcoming seasons. However, this often led to either excess inventory or stockouts. Now, H&M uses AI to analyze factors like online customer behavior, social media trends, and even weather forecasts to predict which clothing items will be popular in specific regions. This allows them to adjust their production and distribution strategies in real-time, reducing excess stock and minimizing markdowns.
AI-powered demand forecasting provides several key benefits:
1. **Improved Accuracy**: AI can analyze larger datasets and more complex variables than traditional methods, leading to more accurate predictions.
2. **Real-Time Adjustments**: AI systems can continuously learn and update their forecasts based on new data, making them more adaptable to sudden market changes.
3. **Enhanced Decision-Making**: With more accurate forecasts, companies can make better decisions regarding production, inventory, and marketing strategies.
By the end of this unit, you’ll understand how AI-driven demand forecasting can help your organization optimize its supply chain, reduce waste, and improve customer satisfaction. In the next lecture, we’ll take a closer look at the tools and technologies that make AI-enhanced forecasting possible.
---
-
12Unit 4, Lecture 2: Tools and Technologies for AI-Enhanced ForecastingVideo lesson
### **Unit 4, Lecture 2: Tools and Technologies for AI-Enhanced Forecasting**
In our previous lecture, we discussed how AI can improve demand forecasting. Today, we’ll explore the specific tools and technologies that make AI-enhanced forecasting a reality. Understanding these technologies is crucial if you want to successfully implement AI in your own demand forecasting processes.
One of the most widely used AI technologies in demand forecasting is **machine learning**. Machine learning models can analyze massive amounts of data, identify patterns, and improve their accuracy over time. But it’s not just about the algorithms themselves—it's also about the quality and variety of data they’re fed. AI thrives on data diversity, from customer purchasing habits to external factors like weather and market trends.
Take **Walmart**, for example. Walmart uses a combination of machine learning and big data analytics to predict customer demand. Their AI system gathers data from thousands of stores, tracking everything from in-store purchases to online behavior. It also incorporates external data like economic indicators and weather patterns. This comprehensive approach allows Walmart to adjust its stock levels in real-time, ensuring that each store has the right products at the right time, while avoiding excess inventory. This system has helped Walmart significantly reduce stockouts and improve overall customer satisfaction.
Another key technology is **natural language processing (NLP)**, which allows AI systems to analyze unstructured data, such as social media posts, customer reviews, and even news articles. NLP can pick up trends and sentiments that might indicate a shift in customer preferences. For instance, a sudden increase in social media posts about a specific product can signal a spike in demand. Companies like **Coca-Cola** use AI-powered NLP to monitor customer sentiment on social media, which helps them forecast demand for new products and marketing campaigns.
Then there’s **cloud computing**, which provides the processing power and storage capacity needed to run AI models at scale. Companies like **Amazon** and **Google** offer cloud-based AI services that make it easier for businesses to implement AI-driven demand forecasting without the need for extensive in-house infrastructure.
Finally, AI can also leverage **deep learning** models, which are particularly effective at recognizing patterns in vast datasets. These models can analyze more complex relationships between variables, improving forecasting accuracy even further. For instance, **Alibaba**, the Chinese e-commerce giant, uses deep learning to analyze customer behavior across its platform. By analyzing millions of transactions and product searches, Alibaba’s AI can predict which products will be popular in specific regions, allowing sellers to adjust their stock accordingly.
By integrating these tools—machine learning, NLP, cloud computing, and deep learning—into your demand forecasting processes, you can gain a significant competitive advantage. In the next lecture, we’ll look at some real-world case studies to see how these tools have helped companies transform their demand forecasting operations.
-
13Unit 4, Lecture 3: Forecasting in Action: AI Case StudiesVideo lesson
### **Unit 4, Lecture 3: Forecasting in Action: AI Case Studies**
In this final lecture of Unit 4, we’re going to explore some real-world examples of how companies have successfully implemented AI-driven demand forecasting. These case studies will highlight how AI has helped organizations optimize their operations, reduce costs, and improve customer satisfaction.
First, let’s look at **Procter & Gamble (P&G)**, a multinational consumer goods company. P&G manufactures a wide range of products, from personal care items to household cleaning supplies. Accurate demand forecasting is critical for ensuring that P&G’s products are always available on store shelves. However, with thousands of products and diverse markets, this was a daunting task. P&G turned to AI to solve this problem. By using AI-powered machine learning models, P&G can now analyze multiple data sources—including sales data, economic indicators, and even local events—to predict demand more accurately. As a result, they’ve reduced stockouts by 15% and optimized their production schedules, leading to significant cost savings.
Another great example comes from **Nestlé**, the world’s largest food and beverage company. Nestlé uses AI to predict demand for its various product lines, from bottled water to baby food. The company’s AI system analyzes historical sales data, weather patterns, and regional preferences to forecast demand in different markets. This allows Nestlé to adjust its production and distribution strategies in real-time, ensuring that each region has the right amount of stock. In one case, Nestlé used AI to predict a spike in demand for bottled water in a region that was experiencing an unusually hot summer. By adjusting their inventory levels accordingly, they avoided stockouts and increased sales during that period.
Now, let’s consider **Zara**, the fast-fashion retailer we mentioned earlier. Zara’s business model is based on rapidly responding to changing fashion trends, so accurate demand forecasting is essential. Zara uses AI to analyze customer data, such as online searches and purchasing behavior, to predict which styles and designs will be popular in the coming weeks. The AI system also monitors social media trends and fashion blogs to get real-time insights into what customers are talking about. This allows Zara to quickly adjust its production and get the right products to stores before competitors, reducing the risk of overstocking or understocking. Zara’s AI-driven forecasting system has been a key factor in its ability to maintain a lean supply chain and minimize markdowns.
Lastly, **Microsoft** has implemented AI for demand forecasting across its vast cloud services. With a customer base that includes millions of businesses around the globe, predicting demand for cloud infrastructure is incredibly complex. Microsoft uses AI to analyze customer usage patterns, business growth trends, and economic conditions to forecast demand for its cloud services. This helps them optimize their data centers, ensuring they have the capacity to meet future demand without overinvesting in infrastructure. As a result, Microsoft has been able to reduce costs while maintaining the flexibility needed to support its rapidly growing customer base.
These case studies demonstrate the transformative impact of AI-driven demand forecasting across industries. Whether you’re managing consumer goods, retail, or cloud services, AI can help you predict customer demand with greater accuracy, improve inventory management, and ultimately enhance customer satisfaction.
As we conclude this unit, it’s clear that AI is not just a tool for optimizing operations—it’s a strategic asset for forecasting future demand. In our next unit, we’ll dive into how AI can improve quality control, ensuring that your products meet the highest standards while reducing the costs of quality assurance.
-
14Unit 5, Lecture 1: Improving Quality Control with AIVideo lesson
### **Unit 5, Lecture 1: Improving Quality Control with AI**
Welcome to Unit 5! In this unit, we’ll explore how AI is revolutionizing quality control processes across industries. Ensuring consistent quality is a critical part of any operation, but traditional methods for quality control often rely heavily on manual inspections and reactive measures. This can be time-consuming, prone to human error, and expensive when issues slip through the cracks.
AI offers a solution that not only increases the accuracy of quality control but also introduces a level of predictive insight that helps businesses address potential quality issues before they become serious problems.
Let’s start with an example from **BMW**, the German automotive giant. BMW uses AI-powered systems to ensure quality throughout its production lines. In one key application, BMW implemented AI to inspect car body frames. The AI system uses computer vision to analyze each frame for defects. These high-resolution cameras scan the surface of the frame, and the AI model identifies minute imperfections that would be almost impossible for the human eye to catch. This automated, real-time inspection process has drastically improved quality assurance, allowing BMW to spot defects early in the production process and correct them before they affect the final product.
AI-enhanced quality control isn’t just about detecting defects; it’s about continuously learning and improving. AI systems get smarter over time as they process more data, allowing them to identify patterns and trends that might indicate a future quality issue. This kind of proactive, data-driven approach helps companies maintain a higher standard of quality while reducing the costs associated with rework and recalls.
But BMW is just one example. In industries ranging from pharmaceuticals to electronics, AI is being used to improve product quality, minimize waste, and enhance customer satisfaction. Over the next two lectures, we’ll dive deeper into the tools and techniques that make this possible and explore real-world case studies of companies that have successfully adopted AI for quality control.
-
15Unit 5, Lecture 2: Visual and Predictive AI Tools for Quality AssuranceVideo lesson
### **Unit 5, Lecture 2: Visual and Predictive AI Tools for Quality Assurance**
In our last lecture, we discussed the significant improvements AI brings to quality control. Today, we’ll focus on the tools and technologies that make AI-enhanced quality assurance a reality. From computer vision to predictive analytics, these tools enable companies to detect issues in real-time and predict potential quality problems before they occur.
Let’s start with **computer vision**. Computer vision involves teaching AI to interpret and understand visual data, such as images and video, in the same way a human would. This technology is particularly powerful for quality control because it allows AI systems to visually inspect products for defects with a level of accuracy and speed far beyond human capabilities.
One great example comes from **Tesla**. In Tesla’s Gigafactories, AI-powered computer vision systems are used to inspect car components during the manufacturing process. These systems can detect defects as small as a fraction of a millimeter—imperfections that would be nearly impossible for a human inspector to catch in real-time. Not only does this ensure a higher level of quality for Tesla vehicles, but it also speeds up the inspection process, allowing production to move faster and with fewer disruptions.
Next, let’s talk about **predictive analytics**. Predictive analytics uses machine learning algorithms to analyze historical and real-time data in order to predict future quality issues. Rather than just inspecting products after they’ve been produced, predictive analytics allows companies to identify potential problems during the production process, ensuring they can be fixed before defects occur.
For example, **General Electric (GE)** uses AI-driven predictive analytics in its turbine manufacturing. The AI system monitors various parameters—like temperature, pressure, and vibration—during production. By analyzing these data points, the system can predict when a component is likely to fail quality standards. If a defect is predicted, adjustments can be made immediately, preventing the issue from progressing further down the line. This proactive approach has saved GE millions of dollars in rework costs and has significantly improved their overall product quality.
But what’s even more exciting is how these tools can be integrated. For instance, **Foxconn**, a major electronics manufacturer, uses a combination of computer vision and predictive analytics to inspect and maintain the quality of their products, such as smartphones and tablets. The AI system scans each product for visual defects while also analyzing data from the production process to predict potential issues. This multi-layered approach ensures that Foxconn delivers high-quality products at scale.
By combining AI-powered computer vision and predictive analytics, companies can achieve a level of quality control that’s proactive, automated, and highly efficient. In the next lecture, we’ll explore more case studies of companies that have successfully implemented AI-enhanced quality control, demonstrating the tangible benefits in terms of cost savings, efficiency, and product quality.
---
-
16Unit 5, Lecture 3: Case Studies: AI-Driven Quality ControlVideo lesson
### **Unit 5, Lecture 3: Case Studies: AI-Driven Quality Control**
In this final lecture of Unit 5, we’ll look at some real-world examples of companies that have successfully adopted AI-driven quality control. These case studies illustrate how AI is not only improving product quality but also reducing costs, minimizing waste, and ensuring customer satisfaction.
Let’s start with **Pfizer**, a global pharmaceutical company. Ensuring the quality of pharmaceutical products is a matter of life and death, which makes accurate quality control absolutely essential. Pfizer has implemented AI-driven quality control systems in its production facilities, particularly for inspecting medications during the packaging process. These AI systems use computer vision to detect defects, such as improper labeling or damaged packaging. Given the high speed of pharmaceutical production lines, manual inspection was previously a bottleneck. Now, with AI, Pfizer can inspect thousands of packages per minute with nearly perfect accuracy. This has drastically reduced product recalls and improved overall production efficiency.
Another compelling example comes from **PepsiCo**, a global food and beverage leader. PepsiCo uses AI to monitor the production of its snack foods. In the past, quality checks were manual, and batches of snacks would sometimes be overcooked or under-seasoned before the issue was caught. Now, AI systems analyze real-time data from the production line, including temperature, cooking time, and even the color of the snacks, to ensure consistency. The AI can detect when a batch is deviating from the optimal cooking process and immediately adjust the settings to correct the issue. This not only ensures that every batch of snacks meets PepsiCo’s high-quality standards but also reduces food waste and energy consumption.
Next, let’s look at **Audi**, the German car manufacturer. Audi has implemented AI-driven quality control systems across multiple points in its vehicle production process. One particularly interesting use of AI is in the paint shop, where AI-powered cameras inspect the paint quality on each vehicle. The AI system can detect even the tiniest imperfections in the paint, such as small bubbles or uneven coating. If an issue is detected, the system immediately alerts the production team so they can correct it before the vehicle moves to the next stage. Audi reports that this system has significantly reduced rework costs and has improved overall customer satisfaction with the final product.
Finally, let’s look at **Intel**, the semiconductor manufacturing giant. The production of computer chips is incredibly precise, and even the smallest defect can render a chip useless. Intel uses AI to monitor the manufacturing process and ensure that each chip meets strict quality standards. AI systems analyze real-time data from the production line, including temperature, pressure, and materials used, to detect any deviations that might impact chip quality. If a problem is detected, the AI system immediately stops production to address the issue, preventing defective chips from continuing down the line. This has helped Intel maintain its reputation for producing high-quality, reliable chips while reducing waste and rework costs.
These case studies show how AI-driven quality control systems can significantly improve the accuracy, speed, and efficiency of quality assurance processes. By adopting AI, companies can not only ensure higher product quality but also reduce costs and improve overall operational efficiency.
As we conclude this unit, it’s clear that AI is a powerful tool for maintaining and enhancing product quality. In our next unit, we’ll shift our focus to workforce management and explore how AI can optimize workforce operations, from scheduling to performance tracking.
---
-
17Unit 6, Lecture 1: Transforming Workforce Management with AIVideo lesson
Unit 6, Lecture 1: Transforming Workforce Management with AI
Welcome to Unit 6! In this unit, we will explore how AI is reshaping workforce management. Managing a workforce efficiently is one of the most complex tasks in operations management, and traditional methods often fall short when it comes to optimizing schedules, tracking employee performance, or predicting workforce needs. AI is now stepping in to transform workforce management, making it more data-driven, predictive, and efficient.
Let’s start with an example from McDonald’s, a company that’s been using AI to streamline its workforce management in its global operations. With over 38,000 locations worldwide, McDonald’s faces constant challenges in staffing its restaurants at the right levels, especially with fluctuating customer demand throughout the day. To solve this, McDonald’s implemented AI-powered workforce management tools that analyze data like historical sales, weather patterns, and even local events to predict peak customer times. Based on these predictions, the system automatically adjusts staffing schedules, ensuring the right number of employees are present during busy periods while reducing labor costs during slower times. This AI-driven approach has increased employee efficiency and helped reduce costs across their global operations.
AI doesn’t just predict staffing needs—it can also help optimize workforce operations by analyzing individual employee performance, improving training, and ensuring tasks are assigned more intelligently. By leveraging machine learning models that continuously learn from performance data, companies can ensure employees are working on tasks that align with their strengths, ultimately increasing productivity and job satisfaction.
Take Uber, for instance. Uber uses AI to match drivers to riders in real time, balancing supply and demand. It also tracks driver performance, using data from rides to help drivers improve their service. This constant feedback loop driven by AI helps Uber maintain a high level of service across its global network.
As we move through this unit, you’ll gain a deeper understanding of how AI can help transform your workforce management processes, improve scheduling, enhance employee productivity, and predict workforce needs with greater accuracy.
-
18Unit 6, Lecture 2: AI for Productivity and EfficiencyVideo lesson
Unit 6, Lecture 2: AI for Productivity and Efficiency
In the last lecture, we explored how AI helps optimize workforce management by predicting staffing needs and matching tasks to employee strengths. Today, we’re going to dive deeper into how AI directly improves productivity and efficiency in the workplace.
Let’s start by discussing Amazon, one of the pioneers in AI-driven workforce management. In Amazon’s massive fulfillment centers, efficiency is key. Every second saved in the process of picking, packing, and shipping items translates to millions in savings. To enhance productivity, Amazon uses AI-powered systems that help optimize employee movements and workflows. For example, AI algorithms track the most efficient paths for employees to take when picking items from shelves. These systems minimize the distance employees travel within the warehouse, saving valuable time and reducing fatigue. This level of optimization allows Amazon to fulfill customer orders faster and more cost-effectively than its competitors.
But AI isn’t just about improving the speed of physical tasks. It can also enhance cognitive tasks, helping employees make better decisions. Deloitte, for instance, uses AI to help its workforce analyze large amounts of data and make quicker, more informed decisions in areas like tax consulting and auditing. AI algorithms sift through complex datasets, identifying patterns and providing insights that human analysts might miss. This not only improves decision-making but also frees up employees to focus on more strategic, high-value tasks.
AI can also improve efficiency by automating repetitive administrative tasks. Take Humanyze, a company that uses AI to analyze communication patterns within organizations. Humanyze’s AI system can identify inefficiencies in workflows by tracking how teams communicate, whether via emails, meetings, or instant messaging. By analyzing these patterns, the system can recommend changes to improve team collaboration and productivity, such as reducing the number of unnecessary meetings or encouraging more direct communication between departments.
Another interesting example is Slack, the team collaboration platform, which uses AI to help streamline internal communications. By analyzing how teams use the platform, Slack’s AI can recommend ways to reduce distractions, such as turning off notifications during focus times or suggesting more efficient ways to organize team discussions. These small changes can have a big impact on overall productivity.
By leveraging AI, companies can improve employee productivity and efficiency at every level, from warehouse workers to knowledge-based roles. In the next lecture, we’ll explore how AI can help predict workforce trends, ensuring you always have the right number of employees with the right skills to meet demand.
-
19Unit 6, Lecture 3: The Future of Work: AI-Driven Workforce StrategiesVideo lesson
Unit 6, Lecture 3: The Future of Work: AI-Driven Workforce Strategies
In this final lecture of Unit 6, we’re going to look ahead at how AI will continue to shape the future of workforce management. We’ll focus on AI-driven strategies that predict workforce needs, helping companies not just react to changes but proactively plan for future demands.
Let’s begin with Walmart, which uses AI to predict workforce needs across its stores. Walmart’s AI system analyzes data such as sales trends, weather forecasts, and local events to predict when stores will experience higher customer traffic. Based on these predictions, the system adjusts staffing schedules and even recommends when to hire temporary workers. This proactive approach ensures that Walmart always has the right number of employees on hand, reducing both labor costs and customer wait times. By using AI in this way, Walmart has increased its operational efficiency and improved customer satisfaction.
Another fascinating example comes from Hilton Hotels, which uses AI to manage its global workforce. Hilton employs over 400,000 people worldwide, and managing such a large and diverse workforce requires predictive planning. Hilton’s AI-driven workforce management system analyzes trends in hotel bookings, travel data, and economic conditions to forecast staffing needs for each of its hotels. The system also tracks employee availability and performance, ensuring that the best staff are assigned to high-demand shifts. By using AI to predict and optimize staffing levels, Hilton can maintain high service levels while reducing labor costs.
AI also plays a critical role in skill development and talent management. In many industries, technological advances mean that the skills employees need are constantly evolving. AI systems can analyze industry trends, identify emerging skill gaps, and recommend training programs to keep employees ahead of the curve. IBM, for example, uses AI to help employees identify the skills they need to develop to stay competitive in their careers. IBM’s AI-powered learning platform provides personalized recommendations for online courses, training programs, and even mentors, based on each employee’s role and career aspirations. This helps IBM maintain a highly skilled workforce that’s ready to tackle future challenges.
AI is also helping companies predict workforce attrition, allowing them to take proactive steps to retain top talent. Microsoft uses AI to analyze employee engagement data, such as feedback from surveys, performance metrics, and even patterns in email communications. The AI system can identify early signs of burnout or dissatisfaction and recommend interventions, such as adjusting workloads or offering additional support. This proactive approach has helped Microsoft reduce turnover rates and maintain a more engaged, productive workforce.
As we look to the future, it’s clear that AI will play a central role in shaping how companies manage their workforces. From predicting staffing needs to helping employees develop new skills, AI-driven workforce strategies will enable companies to stay agile, efficient, and competitive in a rapidly changing world.
In our final conclusion lecture, we’ll bring together all of the insights from this course and explore how you can begin implementing AI across your operations to optimize everything from supply chain management to workforce optimization.
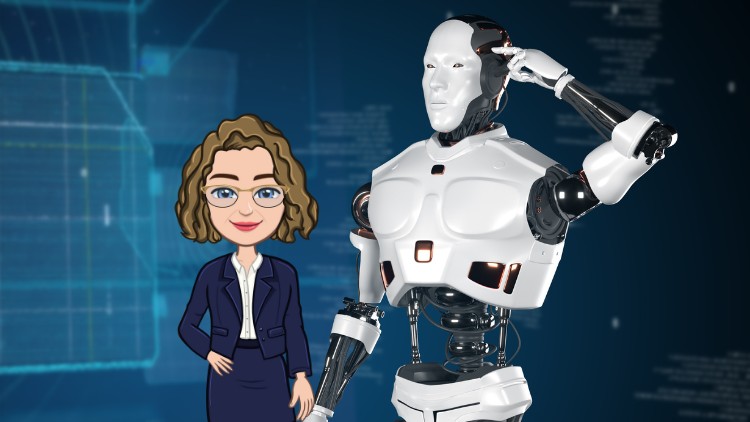
External Links May Contain Affiliate Links read more