Machine Learning using Python Programming
- Description
- Curriculum
- FAQ
- Reviews
‘Machine Learning is all about how a machine with an artificial intelligence learns like a human being’
Welcome to the course on Machine Learning and Implementing it using Python 3. As the title says, this course recommends to have a basic knowledge in Python 3 to grasp the implementation part easily but it is not compulsory.
This course has strong content on the core concepts of ML such as it’s features, the steps involved in building a ML Model – Data Preprocessing, Finetuning the Model, Overfitting, Underfitting, Bias, Variance, Confusion Matrix and performance measures of a ML Model. We’ll understand the importance of many preprocessing techniques such as Binarization, MinMaxScaler, Standard Scaler
We can implement many ML Algorithms in Python using scikit-learn library in a few lines. Can’t we? Yet, that won’t help us to understand the algorithms. Hence, in this course, we’ll first look into understanding the mathematics and concepts behind the algorithms and then, we’ll implement the same in Python. We’ll also visualize the algorithms in order to make it more interesting. The algorithms that we’ll be discussing in this course are:
1. Linear Regression
2. Logistic Regression
3. Support Vector Machines
4. KNN Classifier
5. KNN Regressor
6. Decision Tree
7. Random Forest Classifier
8. Naive Bayes’ Classifier
9. Clustering
And so on. We’ll be comparing the results of all the algorithms and making a good analytical approach. What are you waiting for?
-
66. The Machine Learning Pipeline - Data CollectionVideo lesson
-
77. Importance of Data PrepocessingVideo lesson
-
88. Importance of Feature Selection and Feature EngineeringVideo lesson
-
99. The Machine Learning TerminologiesVideo lesson
-
1010. Introduction to iPython EnvironmentVideo lesson
-
11Important Libraries in PythonText lesson
-
1817. An Intuition on Pandas Dataframe and SeriesVideo lesson
-
1918. Using numpy arrays to create Pandas SeriesVideo lesson
-
2019. Using dictionary to create Pandas SeriesVideo lesson
-
2120. Using a scalar to create Pandas SeriesVideo lesson
-
2221. Series ProcessingVideo lesson
-
2322. Creating Pandas Dataframe from seriesVideo lesson
-
2423. Using lists of data to create a Pandas DataframeVideo lesson
-
2524. Another approach to create DataframesVideo lesson
-
2625. Directly creating a pandas dataframe from numpy arraysVideo lesson
-
3332. Analysis of Linear RegressionVideo lesson
-
3433. Demand vs Price Problem to understand Linear RegressionVideo lesson
-
3534. Implementation of Linear Regression - IVideo lesson
-
3635. Implementation of Linear Regression - IIVideo lesson
-
3736. Visualizing the LBF using matplotlibVideo lesson
-
3837. Why does Linear Regression fail for a classification problem?Video lesson
-
3938. The Sigmoid function in Logistic RegressionVideo lesson
-
4039. The Confusion MatrixVideo lesson
-
4140. Implementation of Logistic Regression - IVideo lesson
-
4241. Creating an heatmap of the confusion matrixVideo lesson
-
4645. Drawing the classification diagramsVideo lesson
-
4746. Introduction to K-Nearest NeighborsVideo lesson
-
4847. Steps in KNN Classification and KNN RegressionVideo lesson
-
4948. Implementing KNN Classification using sklearnVideo lesson
-
5049. Implementing KNN Regression Algorithm in Python - IVideo lesson
-
5150. Implementing KNN Regression Algorithm in Python - IIVideo lesson
-
5251. Introduction to Decision TreesVideo lesson
-
5352. Basic Tree TerminologiesVideo lesson
-
5453. Example 1 for Decision TreeVideo lesson
-
5553.1 Example 2 for Decision TreeVideo lesson
-
5654. Implementation of Decision Tree Algorithm - IVideo lesson
-
5755. Implementation of Decision Tree Algorithm - IIVideo lesson
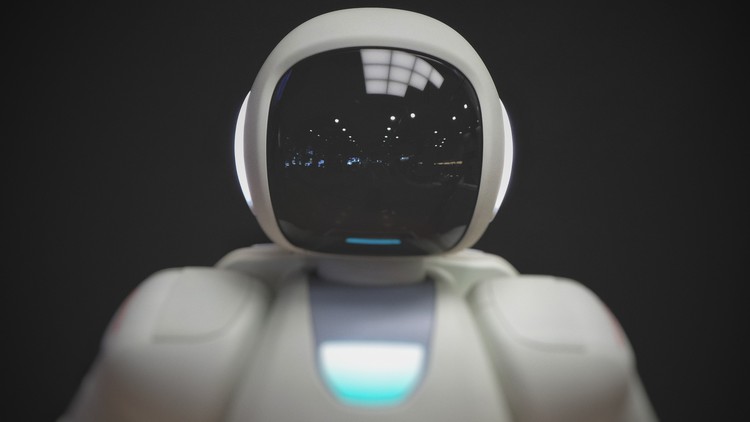
External Links May Contain Affiliate Links read more